Design
On this page we outline how design and other factors can affect metrics and results when conducting eye tracking analytics in XR.
Table of Contents
Calibration
A good calibration is required to get reliable eye tracking data. One way to check the quality of the calibration is to ask participants to look at the stimuli points immediately after the calibration and visually check to see if their gaze is hitting the targets.
In the video you can see an example of a calibration where the user first adjusts the vertical position of the headset, then the distance between the lenses to fit the user’s interpupillary distance (IPD) and is lastly asked to look at a set of calibration points.
The vertical alignment of the headset and the IPD adjustment maximizes the viewing quality by aligning the user’s pupils to the optimal axes of the lenses. The last step is the actual eye tracking calibration. This is needed because the direction of where a person’s eye is pointing is not the same as the direction in which the person is looking. This is due to the fact that the fovea is offset from the back of eyeball by an angle that is different for every person.
Task
Gaze patterns vary greatly depending on the given task. People tend to direct their attention towards the most relevant places needed to complete their current task. This is very clearly shown in the seminal work by Yarbus (1967) on Eye Movements and Vision.
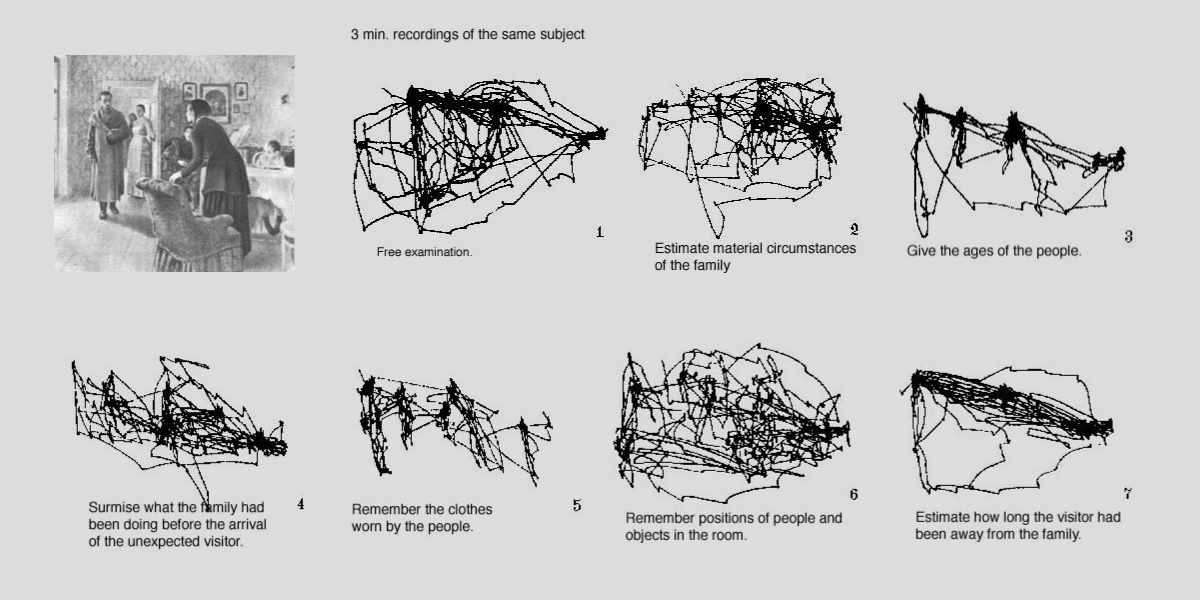
Given this information, it is important to carefully formulate the task you give to participants and to be very consistent when communicating the task to participants.
Scene Design
Realism: To get more realistic results from your study, it usually makes sense to use 3D-models and textures with a realistic level of detail. Our eyes are naturally drawn to areas of high detail, whereas we find it hard to look at something that has few details (for example if you stare at a blank wall).
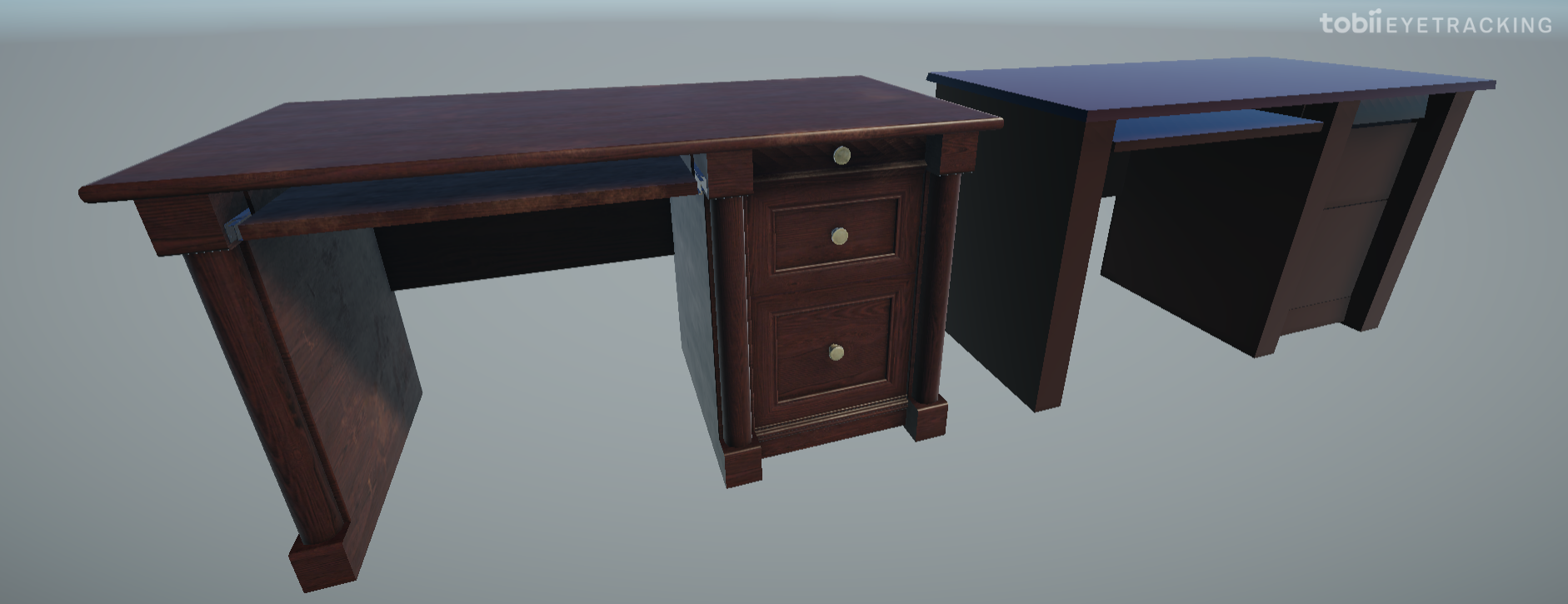
Distractors: In the real world, our eyes are subconsciously drawn to ‘distractors’ that have no relevance to our current task. For example, when training a powerline worker to work on high-voltage power lines, the person will be focused on the pole, lines, tools, etc., but their eyes can be drawn by things that can realistically be expected in such an environment. For example, a bird flying past, a person talking on the sidewalk below, or even something mundane like a yellow bucket in someone’s garden.
Salience: The human eye is naturally attracted to areas of high contrast, vivid color, motion, etc. This phenomena is called Salience. Unrealistically over-salient or under-salient areas will affect your gaze data.
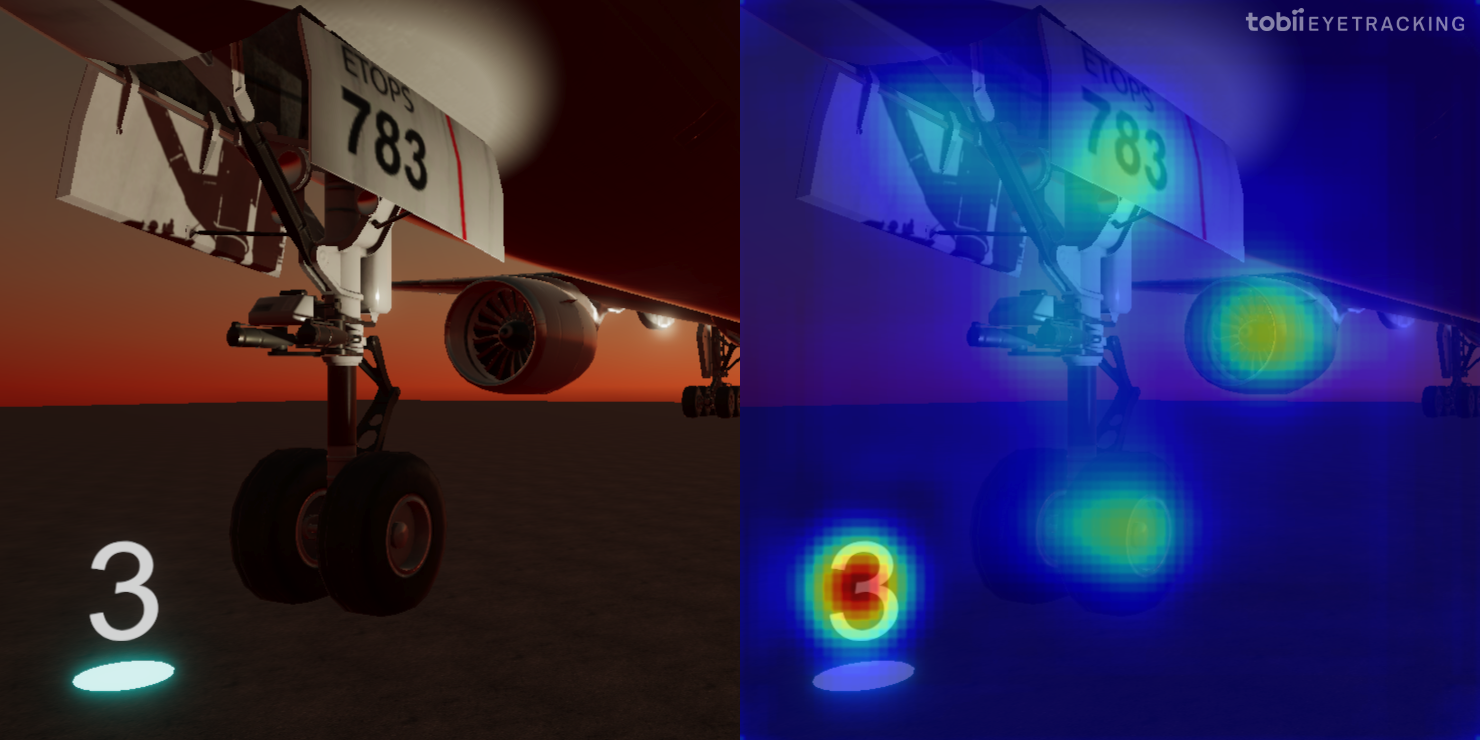
Randomization
In general, people find it easier to look straight forward and at objects at eye level. Therefore whatever object is in that location will get more fixation time than objects in other locations. Usually in the real world we approach things and see them from different angles, making it a good idea to randomize the location of measured objects in a scene for best results.
Keep in mind that randomizing the objects makes it harder to do aggregated visualizations like heat maps.
Locomotion
To move around a scene in VR, the main options are teleportation and smooth movement at walking speed. A downside of teleportation is that it can make the data less representative by for example creating ‘jumps’ when the player is teleporting and can also taint the gaze data because the user is looking at the floor or teleportation visualization. Smooth movement at walking speed eliminates these issues but can cause a bit of motion sickness.
Also keep in mind that VR movement controls will place extra cognitive load on participants, so new VR users may need some time to get accustomed to this.
Additional Notes
Think-aloud: Don’t ask people to ’think aloud’ during the study if you are collecting eye tracking data. When people talk about what they’re thinking, it slows them down and their eyes will fixate on something longer than they would otherwise. They may even stare at an irrelevant object while they talk.
Vision Disorders: Depending on the type of study, subjects can be screened for vision disorders.
Glasses: A large portion of the population wears glasses. Some glasses cause strong glint reflections that don’t track well, and some glasses may not fit in the headset. If the subject normally wears glasses but doesn’t in the study, then they may not look at the things they normally would.
Desensitize New Users: Allow time for people to desensitize themselves from the device if they are new to VR.
Instructions: Instructions needs to be as consistent as possible between participants. Variances in phrasing or intonation can change behavior and change what people pay attention to.
Eye Tracking Awareness: Being aware that the headset has eye tracking can make people overly aware of where they look. One strategy is to not mention that the headset tracks your eyes, until afterwards.
Validation: It can be harder to validate VR results when a real-world scenario is studied but VR allows for repeatable studies in a controlled environment for use cases with high risk or cost. For AR, it’s easier to tie the results to the real-world but it’s harder to map the data and you don’t get the above mentioned advantages.
Pilot Study: Conducting pilot studies can help you catch flaws in the design early on and may allow you to avoid missing importing factors before collecting large amounts of data.